Target marketing through data science and how to build a career around it
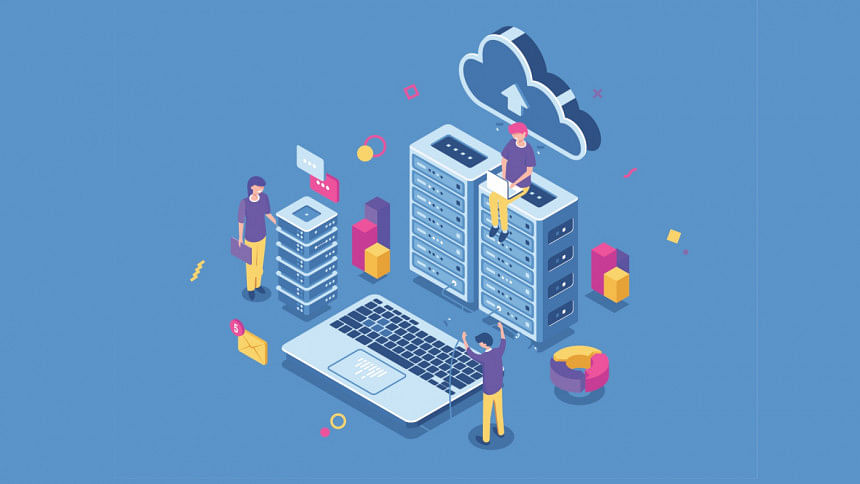
Let's bust some myths about data science first. While most people think it's all about machine learning, AI, deciphering codes and things like that, it's not the case most of the time. The misconception prevailed and still prevails to some extent because most data scientists were hired by companies for analytical jobs. In reality, data science is not really about deciphering the codes, rather it's more about using data for creating the maximum positive impact for a company or organisation. Data science is a combination of computer sciences and data mining, which is defined by the Journal of Data Science as 'almost everything that has something to do with data: collecting, analysing and modelling. Yet the most important part is its application- all sorts of applications'. The application bit includes machine learning along with many other important aspects, but that somehow overshadows all the other aspects of data science.
From a target marketing perspective, the job of a data scientist is to gain insights on the products, craft product recommendations and use data to improve existing products. Making complicated models and data visualisations come way later in the list of priorities. For example, companies like Google, Facebook and Netflix don't need to rely on the complex data analysis, rather they look for data scientists who can solve the most complex of problems using data and work as a strategist for their company. Same goes for many other local companies in the e-commerce market. Market research is a key aspect that needs the work of a data scientist. A data scientist can work through an extensive range of data and find the factors that might improve the sales, or even forecast the demands and expected revenue of a certain product. How people react to certain advertisements on social media can be recorded and then interpreted to forecast the demand or sales that the product is expected to generate. Successful target marketing is a key reason for which companies often rely on data scientists these days.
While companies with more resources can have a better system in terms of work distribution and employ more people specialising in each aspect of data science, they prefer having Master's or PhD degree holders on board for more advanced and technical aspects of the job, i.e. jobs involving AI and rigorous machine learning. People who are eligible for pursuing a career in data science represent a variety of majors from different faculties, such as- computer science, software engineering, environmental engineering, systems and management engineering, economics, statistics, mathematics, etc. However, undergraduate freelancers can self-train themselves or take up courses to develop the skills needed to make a career out of it, and can contribute to the more basic aspects that the companies need, such as setting up databases, working with the analytics, building up the metrics, running experiments and developing simple algorithms. There are vast career opportunities in this sector for people with different skill levels. However, there are some basic steps that everyone wanting to pursue a career in data science should follow.
Learning SQL and data metrics
SQL is considered to be the easiest language for building up the basic infrastructure of a database. It is widely used by people employed in jobs that involve business intelligence. A good knowledge of data metrics is necessary for developing the build of a good product, which will determine the success of an endeavour.
Learning other software languages
Expertise in IPython, R, Excel and Tableau is needed to develop better skills in manipulating, visualising and interpreting data. What matters more than technicality is the ability to communicate and interpret the data in a better and easier way. In the end, it really doesn't matter which software you use as long as you are fast and effective in communicating and interpreting the findings.
The experience of an internship
After developing a certain set of skills, it is important for anyone wanting to pursue a sustainable career in data science to get a proper internship experience. This can help you prepare yourself for the real challenges you might face while working as a professional data scientist. It can also increase your ability to spot the implications of your findings to a great extent, which can effectively increase your chances of landing greater career opportunities in this sector in the future.
Developing communication and presentation skills
Communication is crucial no matter which sector you work in. Speaking about this particular sector, the entire point of data science is to communicate and interpret the findings that come through rigorous analysis. Improving your writing and Power Point skills IS important as well, as good presentation skills can take you a long way ahead.
Nahaly Nafisa Khan is an Economics major, currently studying in DU. She procrastinates a lot, yet is obsessed with meeting deadlines. You can reach her at [email protected].
Comments