How startups can make the best use of data
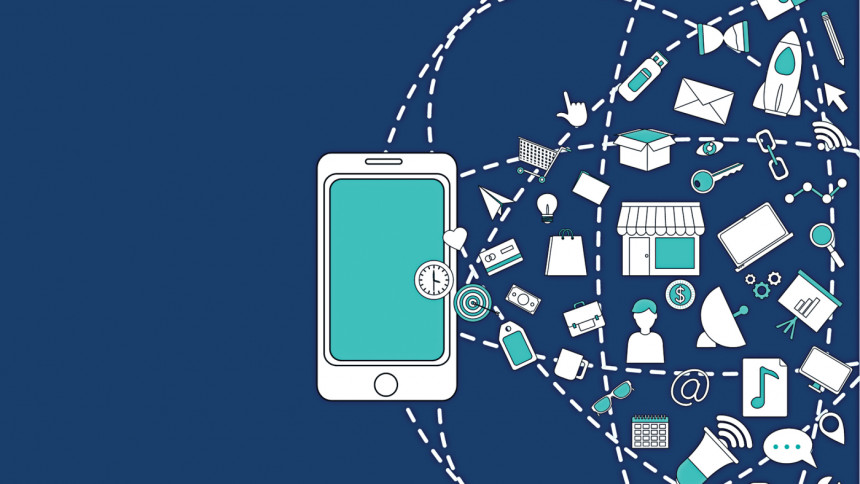
We live in the age of data. Today, as I write this article nearing midnight, almost 2.5 quintillion bytes of data have been generated globally. That is 25 followed by seventeen zeros. And we in Bangladesh are not much behind in this stream of data generation. A good back of the envelope calculation will be that since almost two percent of the world's population live in Bangladesh, and assuming that one in every 100 persons in the country has access to devices that generate data, we will be generating 500 terabytes (TB) of data per day. And I am making some very conservative estimations here. That's more than the highest storage space available in personal computers or hard disks (which max up to 20 TB).
As a result of this predominance of data everywhere, many successful business names today have grown by leveraging data. Companies such as bKash have utilised insights from data to make sure that their retailers are working properly, to identify the wallet sizes in different regions, and to predict the right amounts of cashbacks that incentivise customers to use the mobile wallet. Pathao has used massive amounts of data and their unique graph algorithm (a data science tool) to match the rider and the user which has helped them compete against global giant Uber. Even brick and mortar organisations such as Unilever and British American Tobacco are investing heavily on setting up their data teams and making the right use of data to increase revenue.
But for a startup, making the best use of data can be challenging. There are quite a lot of issues to consider – how and where to obtain the data? How to set up a data team? How much to invest? When? How to scale up? Which tools and platforms to use? And these challenges are exacerbated by the fact that startups are short on funds, always under pressure from investors to show positive return on investment (ROI), and be as lean as possible.
Therefore, the first issue really is to determine how and where to obtain the data. But that is honestly the easier part. Most business models today are either online or involving services that are digital or connected to social media. A startup can chart its whole process of doing business, from the very first steps, till the service or the product is delivered to the customer, and identify exactly the points where they can get data. For example, in the case of a company that provides ride services to university students and professionals, the data can be in the form of what routes are being requested in the company's website, its social media channels and its hotlines, what are the existing routes, how many customers are using the existing routes, who are the recurring customers, how many vehicles are operating in each of the routes, what is the spare capacity, etc. And this is where the company needs to make another major investment – building a data culture, where the focus is always on evidence driven decision making. Everyone in the company should at least be aware of how valuable data is for decision making, to support their hunches, experiences, and sparks of brilliance, and also be on the lookout for which data points they can obtain to connect the company's performance. And this culture needs to be driven from the very top – startup founders need to instil the value of data. This has successfully been accomplished by visionary startup founders such as Reyasat Chowdhury of Shuttle, or Oli Ahad of Intelligent Machines Limited, both of which have reaped significant benefits from data utilisation.
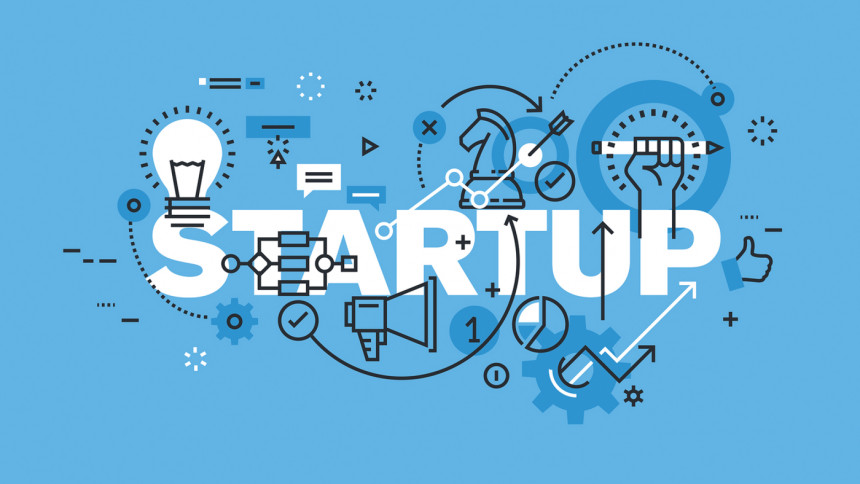
The second issue is to understand when the time is right to invest in a data team. This is a more difficult challenge to handle as there is no "one size fits all" solution to this. If the startup's core business model is to generate insights from data, or utilise data, such as that of Pathao, then the data team is the first team to develop and train up, with an experienced data leadership, a chief data officer (CDO) or a chief technology officer (CTO). But there are many startups whose business models do not only revolve around data. For them, to initially invest on a dedicated data team led by CDO/CTO might be prohibitively expensive. In such cases, the startup can approach the issue from a different angle, channel it's prime resources and priorities launching a minimum viable product (MVP), and start generating cash flows as soon as possible while keeping in mind what nature of data is generated at every step and store that data. These storages will not need much of an investment, but some investment would be required to set up means of collection. If the startup is unsure of what data can be helpful, or how to set up the collection, they can hire external consultants and advisers to help step up the collection and data management. But when the startup reaches a certain stage where there is a recurring revenue flow, and the MVP is launched and being accepted in the market, that should be the ideal time to set aside some of the revenue to build a good data team.
Let me elaborate more on how a company should strive to build a good data team. I have seen over the years many attempts at creating data teams and drives by businesses to be data driven, only to yield disappointing results. One key reason for this is the lack of understanding by the organisation's leadership of what data driven decision making is, and how it can help them. A common approach is to hire someone from a technical background such as Computer Science or Electrical and Electronic Engineering (EEE), and then ask them to start processing the data that has been accumulated or start building databases and reporting structure. I am confident that any technical graduate from a good engineering school in Bangladesh can do an incredibly good job on this, but in the longer run, the management fails to realise the benefit of it, because what they get is a report on their table, or a dashboard on their laptops summarising the basic findings, which they see and don't act on.
To get the right team and have them deliver profitable changes to the startup, much more is needed. It starts with the organisation's leadership understanding what the data can do for them, and building data literacy at the topmost level of the organisation. This does not need to be technical, but the senior management of the company needs to understand what data they have, how they can gain a competitive advantage with this data, and what nature of insights they are looking for. This can be achieved with the help of expert consultants or trainers, to learn of sources of data and methods (tools and techniques) of driving decisions and strategies using data. The next step is to appoint a data team lead – someone who understands the nature of the business the startup is in, someone with experience in managerial positions or leadership positions, with good business acumen, and a good understanding of the technical concepts of data science. In short, the startup needs to hire a "data strategist," someone who not only can code and crunch numbers, but can drive strategies using the output from the data tools and techniques. Then the focus should be on hiring technical talents for data storage, pipelines, and reporting. Many times, the organisation's drive towards data transformation fails due to missing out on these two crucial steps – data education of its leadership and hiring a data strategist.
The rest of the issues are more technical in nature and can be handled when the organisation has invested in a capable data strategist and team.
Comments