Policies without proper data are doomed to fail
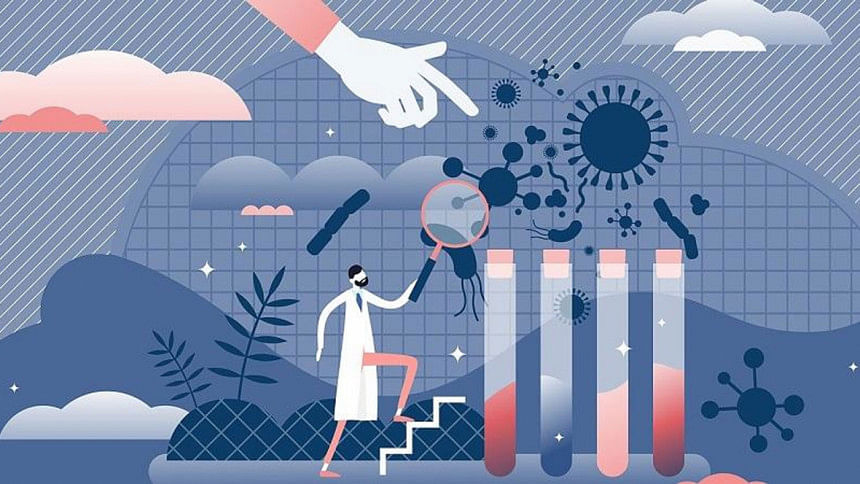
For all of us who are passing through this pandemic, whether sheltered in our cosy homes or crummy shanties, or on the street and markets serving people, or at the hospitals caring for the ailing patients, one thing is clear: We are not on the decision-making side. We can chat-cry-fight or eat-pray-live while uncertainty looms over our overcast horizon. Do not get us wrong, the decision-makers are in disarray as well, desperately searching for evidence and advice from those who claim to have done considerable research. Scholarly publications and suggestions directly or indirectly impact short-mid-long term policies of the governments.
Recently, two scholarly articles regarding Covid-19 and the issue of monetising human life came to our attention. The first one is "Should Low-Income Countries Impose the Same Guidelines as Europe and North America to Halt the Spread of Covid-19?" by Barnett-Howell and Mobarak (2020). The researchers conducted a cost-benefit analysis (CBA) and predicted mortality from the five policy scenarios (adopted from Walker, Whittaker, and Watson et al., 2020), and measured the economic value of avoided mortality from mitigation policies for several countries, using Viscusi and Masterman's (2017) country-specific value of statistical life (VSL) estimates.
Their research came with findings like—"Without mitigation policies, Covid-19 still imposes a large welfare cost above 130 percent of GDP in rich countries like the United States and Japan. In contrast, due to the lower predicted mortality rate, the losses in India, Bangladesh, Pakistan, Nigeria, Nepal in the unmitigated scenario are about 50-60 percent of their own (lower) annual GDP ". The article also stated that "…Imposing social distancing yields a very large welfare improvement in rich countries: Equivalent to 59 percent of US GDP. However, the same policy of social distancing increases estimated welfare in Bangladesh by only 14 percent of its own lower GDP."
The paper claims to have identified lower estimated benefits of social distancing and social suppression in low-income countries and states that the difference gets larger if more suppressive policies are taken. The second work is an article in Prothom Alo (May 28, 2020) by Moshahida Sultana, in which the author briefly introduces the conceptual limitations of VSL. She rightly points out the problem of comparing statistical life against actual human life and the fact that society's attitude of monetising human life varies significantly across countries, which are often ignored in VSL calculations.
Now, these two works provide an excellent platform to discuss this issue further. It may be true that when policymakers take decisions like withdrawing lockdown or social distancing, they explicitly or implicitly put a monetary value on human life and compare it with the projected economic loss. Therefore, in such circumstances, the most critical issue is to ensure that the mechanism used does not systematically devalue the life of the poor or sick. Introducing models such as VSL abruptly in the pandemic situation has faults in two dimensions—firstly, if it is calculated repeatedly we are bound to get results with extreme uncertainty and secondly, if data used are not current and cherry-picked to serve a purpose, the model will back policies that may have a detrimental effect.
So, what is value of statistical life? In simple words, VSL is the amount of money that a person or society is willing to pay to save one human life. However, this human life is not any specific person's life but an anonymous life (one life in a million). Therefore, it is referred to as statistical life. Now the very first question arises, if the government wants to use VSL, what is the value of a Bangladeshi statistical life?
There are several studies in the Bangladesh context that utilises different methodological approaches and provides different monetary values of human life. Using the benefit transfer method (i.e., extrapolating the estimates obtained from meta-analysis of surveys conducted in certain countries to calculate the VSL for other countries), Viscusi and Masterman (2017) show that Bangladeshi VSL is USD 205,000. According to Miller (2000), VSL lies between USD 30,000 to 10,00,000. Robinson et al. (2017) found it to be USD 142,709. According to Mahmud's (2009) estimate, VSL for rural Bangladeshis ranges from USD 1,498 to 2,455. In another study, Mahmud and his colleagues (2019) estimated VSL for urban Bangladesh ranges from USD 17,480 to 22,463.
The values vary significantly because VSL calculates the willingness to pay (WTP) to avoid potential risk, either by stated preference or revealed preference (i.e., preference observed from individual's behaviour) method. Individuals do not always fully understand the level of risk, especially in the developing country context. Hence, the value of WTP depends highly on the survey method. Moreover, in the benefit transfer method, the VSL calculation is based on the assumption that the income elasticity or responsiveness of VSL is constant and equal across the country of interest, which is a very weak assumption to hold. Income elasticity of VSL varies with income level, wage, and age. Furthermore, heterogeneity in personal characteristics (income, wage, ethnicity etc. factors) affects the marginal WTP for reducing fatal risk.
Secondly, the effect of the time dimension on VSL should also be taken into account. Without an up-to-date VSL estimate, the decision-makers cannot know the responsiveness of VSL to income transition in recent years. Furthermore, the risk of a job also may vary substantially over time. A single or backdated VSL estimate should not be used to estimate the welfare loss of a country and an investigation on the cross-year VSL change should be conducted to gain accurate policy insights.
Thirdly, both in the VSL calculation and cost-benefit analysis, researchers rely only on the value of gross domestic product (GDP) or gross national income (GNI) of the country of interest. A strong alternative view has emerged in recent years, which argues that such analysis should be based on a wellbeing framework instead. Now, one can argue that GDP or GNI are useful measures of economic activity and are highly correlated with wellbeing. While dealing with a pandemic, can we ignore wellbeing—health, environmental issues, quality of life—the parameters that are not part of the equation? Countries like New Zealand have started to use the wellbeing framework to calculate the national income besides the conventional indicators.
Lastly, CBA is a useful tool for microanalysis, like evaluating specific micro intervention programmes. But it has its limitations, and without considering them, it can provide serious questionable outcomes, especially in case of macro analysis. There are some examples of this in the literature regarding the outcome of CBA analysis. For instance, in a study, Viscusi (1994) concluded that states had saved money as their citizens who smoked died early. Another example that is relevant for developing countries is how a plethora of water and sanitation intervention studies evaluating the fatal cases of diarrhoea, claim that time-saving is a more dominant component than the health benefits of such programmes.
However, as Hunt (2011) argues, in most cases, these studies only calculate the loss of lifetime earnings and wholly ignore the pain and suffering component of the welfare cost. Such welfare cost is often higher than the cost of illness. So how can these models be made somewhat useful? Cost-benefit analysis should embrace a wellbeing framework rather than conventional indicators. Surveys need to be contextualised, current and carefully designed. Most importantly, backdated data should not be used.
This pandemic has shown us how little we know about our people, our health system, and our overall socioeconomic condition. Do we have any systematic mechanism to accumulate information on how much the economic activity across different sectors and regions may have reduced due to the Covid-19 lockdown? Do we know how much of the economic downturn is driven by demand shock and by supply shock? Do we know if it is a persistent economic fall? Do we have accurate information on which group of people are most affected? Do we have real information about people's mobilisation patterns? Lack of data stifles our ability to make decisions as required, and compels us to take abrupt interventions fraught with uncertainty. The lesson we learn is simple: the government should take a data responsive strategy to make informed decisions when an immediate response is required.
Policy without proper data ignores and excludes the life of the poor while policy based on imperfect data underestimates. We could argue in favour of cost-benefit analysis using the statistical value of life if updated data with an accurate model could be employed. Alas! That is not the case. So we urge others to think twice before considering such research conclusions and hope that the government will take effective measures in reducing the data gap to initiate a genuine Pareto improvement in the policy response mechanism.
Amin Masud Ali, PhD is Associate Professor at the Department of Economics, Jahangirnagar University, Bangladesh, and Moinul Zaber, PhD is Senior Academic Fellow at United Nations University Egoverment Operating Unit, Guimares, Portugal. Emails: [email protected], [email protected].
The views and opinions expressed in this article are those of the authors and do not necessarily reflect the official policy or the opinions, beliefs and viewpoints of their respective institutions.
Comments