The promise and pitfalls of AI in clinical reasoning
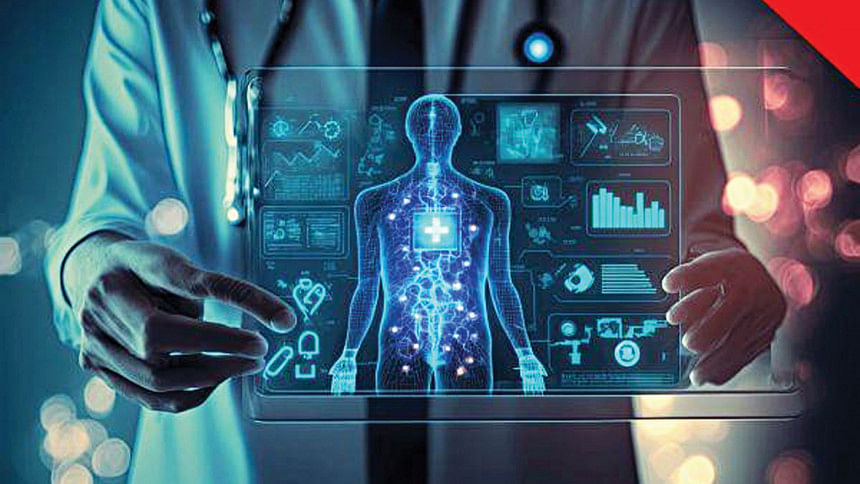
Large language models (LLMs) like GPT-4 and Gemini-1.0-Pro are revolutionising clinical reasoning, demonstrating expert-level diagnostic capabilities. However, these tools are not without flaws, mirroring the cognitive biases that challenge human decision-making. Recent studies highlight both their potential and limitations in clinical practice.
One study examined the impact of GPT-4 on clinicians' diagnostic accuracy by presenting complex vignettes to 50 physicians randomised to use either standard tools or standard tools plus GPT-4 (JAMA Netw Open 2024; 7:e2440969). GPT-4 outperformed human groups when used independently but did not enhance clinicians' performance when combined with standard tools. This underscores the need for training to maximise AI's effectiveness in real-world contexts, which involve complexities beyond written cases.
Another study evaluated whether LLMs exhibit cognitive biases, testing GPT-4 and Gemini-1.0-Pro with clinical scenarios designed to expose flaws (NEJM AI 2024; 1:AIcs2400639). Results revealed biases such as the "framing effect," where treatment recommendations varied based on how survival or mortality was presented. Similarly, the "primacy effect" influenced AI's diagnostic prioritisation, while "hindsight bias" affected judgements on past care.
Interestingly, AI's biases were sometimes greater than those observed in human clinicians. Experts recommend clinicians use critical questioning strategies to challenge AI-generated conclusions, such as asking for alternative hypotheses or evidence against a diagnosis.
As LLMs become integral to healthcare, rigorous evaluation and thoughtful integration are essential to mitigate risks and harness their potential for improved patient outcomes.
Comments