Data Analytics: What it is and why it matters
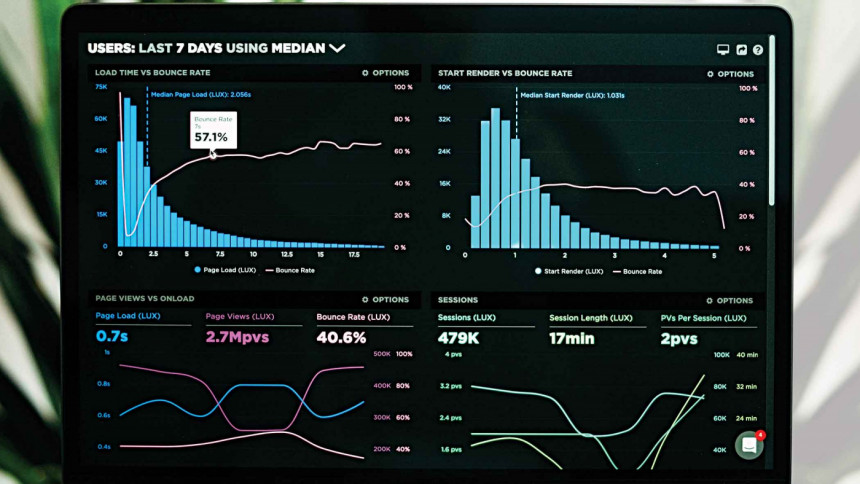
To drive productivity, efficiency and revenue growth in any sector, data analytics plays a significant role by extracting insights from data. Using modern technology in different businesses and corporations has generated huge amounts of valuable information, leading to a boom in the data industry in recent times. An assortment of various applications, tools and methodologies collectively known as data analytics can help make sense of this vast volume of data. Khan Muhammad Saqiful Alam, Program Manager, Trust and Safety, South Asian Region, TikTok, provided an overview of data analytics in the webinar titled "Data Analytics for Budding Economists", organised by Economics Study Center (ESC), University of Dhaka on June 11, 2021.
What is data analytics?
Data analytics refers to some qualitative and quantitative methods that analyse datasets, observe trends and actions, and make evidence-based and strategic decisions. The process is a good way to observe behavioural patterns among many other things. Note that data science and data analytics are not the same. Data science has 5 aspects: designing and automating data collection systems, storing data by cloud computing, data processing, data analysing, and communicating the outcomes of the analysis to the stakeholders. Mostly the data analysing part of data science is covered by the field of data analytics
How do companies use analytics to make profits?
A real example of using data analytics for business operations is Pathao. It uses graph algorithms that connect riders to people seeking rides in the shortest distance at the lowest price possible. Pathao uses data analytics to analyse vast volumes of datasets regarding location, consumers' behaviour and demand and act according to the findings. Another example is Amazon. Amazon started as a bookstore which is now the world's leading online retailer. A major part of its success is due to its up-selling through both 'Frequently Bought Together' and 'Customers Who Bought This Item Also Bought' sections. Using data analytics, Amazon finds your behavioural twins and shows relevant things in these sections that may interest you when you use their site. It also records your activities to analyse behavioural patterns.
Sources of data in data analytics and tools to record them
In the process of data analytics, data can be in any structured or unstructured form such as text, image, audio, video, and so on. While working with web data, one can use a free analytics program named 'Google Analytics' (GA). One drawback of web data is that you need to own the website to track its activities. But if the respective authority gives access to modelling more support to you in your analysis, you can view their GA data. TalkWalker, a social media data aggregator tool, helps in analysing social media data. It provides real-time insights by measuring sentiments, brand health and the performance of different brands. There is another type of data called observational data that records what people generally do. For example, a super shop can observe their customers' behavioural patterns through CCTV cameras and rearrange the aisles according to them.
For text analysis, you can use 'Voyant Tools'. It can be used to identify broad themes or most common themes that have surfaced in Focused Group Discussions (FGDs) or interviews in qualitative research. Web scraping tools, such as Octoparse, enables both experienced and inexperienced users to extract bulk information from websites. These tools can be used by coders and non-coders alike!. Just enter the particular URL link, click on the target data for extraction and get the data that you need. The concept of A/B testing, a form of Randomised Control Trial, can come in handy to establish causality and measure the real impact of any decisions made.
Methods of analysing these data
Besides many new tools in collecting some fascinating types of data in the age of the internet, businesses, economics research and any other decision making can benefit significantly from the new methods of analysing these data. Besides the commonly used Linear Regression, the analytics tools and applications can also help with non-linear regressions, helping in modelling more complex relationships and making predictions better. And analytics introduces new types of methods as well. Unsupervised learning methods such as K-Means clustering can help divide and categorise data into small segments and find patterns/groups among the segments. This help when the behaviour or nature of relationships in the data varies across segments. Other than that, Decision Trees and Recursive Partitioning tools can help model data that does not follow any simple relationship patterns. These models have been famously used to identify any rules that were used to load the lifeboats when the Titanic was drowning! To sum up, it's a brave new world for the use of these new methods to answer more and more fascinating questions!
Comments