Data-driven lending solution for Bangladeshi banks
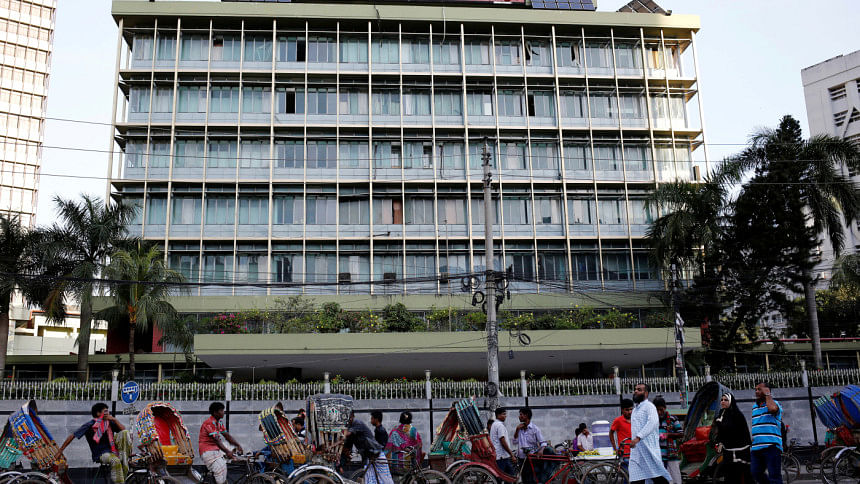
In Bangladesh, the process of securing a loan from most banks and non-bank financial institutions (NBFIs) feels like a maze. A small business owner walks in with a mountain of paperwork, a salaried professional submits their home loan application with fingers crossed, and a credit officer begins the tedious task of checking documents and preparing loan appraisal reports. This cycle plays out day after day, with loan decisions made based on outdated risk management practices. Weeks, even months, pass, only for many of these hopeful applicants to face rejection, not because they lack potential, but because the system never truly understood their financial story.
This situation is the silent crisis of our banking system. In a country where the middle class is expanding and millions of small businesses drive the economy, the financial system still struggles to meet their needs. Why? Because credit risk management has not evolved with the times. But there is a solution, and it lies in reimagining the way we assess risk through the lens of data.
Bangladesh is a country of 170 million people. One in four now belongs to the middle class, and that number is growing thanks to rapid urbanisation and digital adoption. This upward shift is opening up big opportunities, especially in retail banking.
Meanwhile, around 10 million CMSMEs (cottage, micro, small, and medium enterprises) comprise 90 percent of industrial units and account for 80 percent of industrial employment. Despite their vital significance, access to finance remains limited simply because they don't have formal documentation, collateral, or traditional credit histories.
This is not a capacity problem. It is a visibility problem. And that is where data-based risk management can change everything.
Data-driven credit analytics offers a powerful alternative to the old way of credit risk management. Instead of relying on a rigid set of forms and a credit officer's intuition, financial institutions can use machine learning, an algorithm trained on years of data from past borrowers, to evaluate the creditworthiness of a potential borrower. This model does not just look at income or collateral; it captures patterns in mobile transaction records, utility bill payments, digital wallet activity, geo-location data and other alternative indicators to assess how a borrower earns, spends, and saves. For CMSMEs, it means analysing business turnover, supplier relationships, cash flow position, and other relevant data points. Within minutes, a machine learning model assigns a credit score rooted in actual outcomes, not outdated assumptions.
The need for smarter risk assessment has never been more urgent. As of December 2024, non-performing loans (NPLs) in Bangladesh reached an all-time high of 20.2 percent of total outstanding loans. The banking sector remains fixated on a small number of large classified loans while overlooking thousands of creditworthy individuals and small businesses. As Bangladesh aims to rebuild its banking system, leveraging data analytics is pivotal for its banking sector to remain competitive and resilient.
One of the greatest advantages of data-driven risk management appears in the post-disbursement phase. In a traditional risk management approach, financial institutions only learn about borrower distress after it is too late, when a payment is missed or a default occurs. By then, recovery becomes costly and complicated. On the contrary, real-time data analytics allows for proactive monitoring. If a borrower's transaction volume drops or their repayment behaviour changes, analytics systems can flag the risk immediately. Financial institutions can step in early with a reminder and the right recovery plan.
Historically, banks and NBFIs avoided small loans because of the high cost of customer acquisition and supervision. But digital technology is flipping that logic on its head. With the right data infrastructure, financial institutions can automate most of the credit lifecycle: from application to approval to monitoring. This reduces operating costs and makes small-ticket retail and SME lending not just viable, but profitable.
Beyond traditional financial product offerings, data analytics is also opening doors to embedded finance, a model where credit is offered directly through platforms people already use. Think of a ride-hailing driver getting a loan from the app they work for. Or an online seller receiving instant credit based on their digital sales history. According to a report published by ResearchAndMarkets.com, embedded finance is projected to grow at a 48 percent annual rate from 2024 to 2029, with revenues expected to hit $5.8 billion. However, to tap into this opportunity, financial institutions need to shift from product-based offerings to ecosystem-based thinking. That means building APIs, investing in cloud-based infrastructure, and most importantly, using data as the foundation of all credit decisions.
The good news is that the transition to data-based risk management doesn't require a complete overhaul. Most banks already have years of valuable data sitting in their systems. Building a scorecard model using that data to predict default risk is both doable and cost-effective. Integrating modern loan origination systems (LOS) with existing core banking platforms is a proven path. What is needed now is a mindset shift: from fearing risk to understanding it, from gatekeeping to enabling and from guesswork to insight.
Several forward-thinking banks and NBFIs in Bangladesh have already taken this step. By integrating a robust LOS with their legacy core banking platforms, they have successfully adopted data-driven risk management systems. These institutions are thriving, benefiting from faster loan processing, more accurate credit assessments, and reduced default rates.
Imagine a Bangladesh where a woman running a home-based clothing business can get a small loan based on her online sales, where a local grocer in Bogura is evaluated not by collateral but by years of consistent supplier payments and sales turnover, and where a ready-made garment worker can access an affordable home loan to construct a semi-pucca building on the outskirts of Dhaka, in Savar. That future is not far off. It is waiting at the edge of data and the financial institutions willing to harness it.
Financial institutions that lead this shift will not only find new markets and revenue streams. Surely, they'll build something far more valuable: trust, inclusion, and long-term resilience. The future of credit risk management is not about rejecting loans. It is about knowing who to trust and letting data tell the story.
Shah Jalal is a risk management professional working at a non-bank financial institution. He can be reached at [email protected].
Views expressed in this article are the authors' own.
Follow The Daily Star Opinion on Facebook for the latest opinions, commentaries and analyses by experts and professionals. To contribute your article or letter to The Daily Star Opinion, see our guidelines for submission.
Comments